Do you use EC 2?
What is EC 2?
Amazon Elastic Compute Cloud is a part of Amazon.com's cloud-computing platform, Amazon Web Services, that allows users to rent virtual computers on which to run their own computer applications.
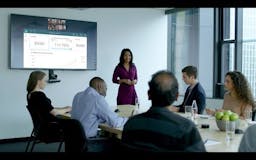
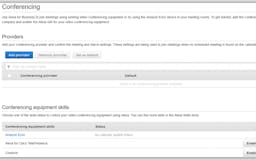
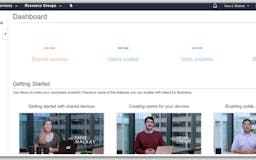
Recent launches
Amazon Web Services (AWS)
Amazon Web Services (AWS) is the world’s most comprehensive and broadly adopted cloud, offering over 200 fully featured services from data centers globally.
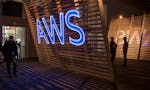
Alexa for Business
Alexa for Business is a new service that enables businesses and organizations to bring Alexa into the workplace at scale.
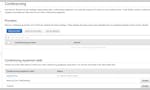